How Can You Handle Imbalanced Datasets in Machine Learning?
In the world of Machine Learning, Artificial Intelligence, Data Mining, and Predictive Analytics, dealing with imbalanced datasets is a big challenge. These datasets happen when one class has much more data than the others. This leads to biased models and wrong predictions. Examples include fraud detection and disease diagnosis.
Handling imbalanced data is key to making Machine Learning models work well. Techniques like oversampling, undersampling, and using class weights can help. These methods improve how well the model performs.
Data scientists and Machine Learning practitioners can tackle imbalanced datasets. By using the right techniques, they can build models that accurately predict both common and rare classes. This is crucial for creating reliable Machine Learning solutions that impact many industries.
Understanding Imbalanced Datasets and Their Impact
In machine learning, datasets often have an uneven mix of class labels, known as imbalanced datasets. This happens when one class, the majority, makes up most of the data. The minority class, however, is much smaller. This imbalance can greatly affect the performance of Deep Learning, Neural Networks, and Natural Language Processing models.
What Defines an Imbalanced Dataset
An imbalanced dataset has an uneven mix of class labels. It’s mildly imbalanced if the minority class is 20-40% of the data. It’s moderately imbalanced at 1-20%, and extremely imbalanced at less than 1%.
Common Examples of Data Imbalance
Imbalanced datasets are common in many areas. For example, in credit card fraud detection, rare disease diagnosis, and anomaly detection in manufacturing. Here, the majority class, like non-fraudulent transactions or healthy individuals, is much larger than the minority class, like fraudulent transactions or rare diseases.
Challenges in Classification Tasks
Imbalanced datasets make classification tasks hard. Machine learning algorithms often focus too much on the majority class. This neglects the minority class. As a result, models can be skewed, leading to poor performance and misleading metrics.
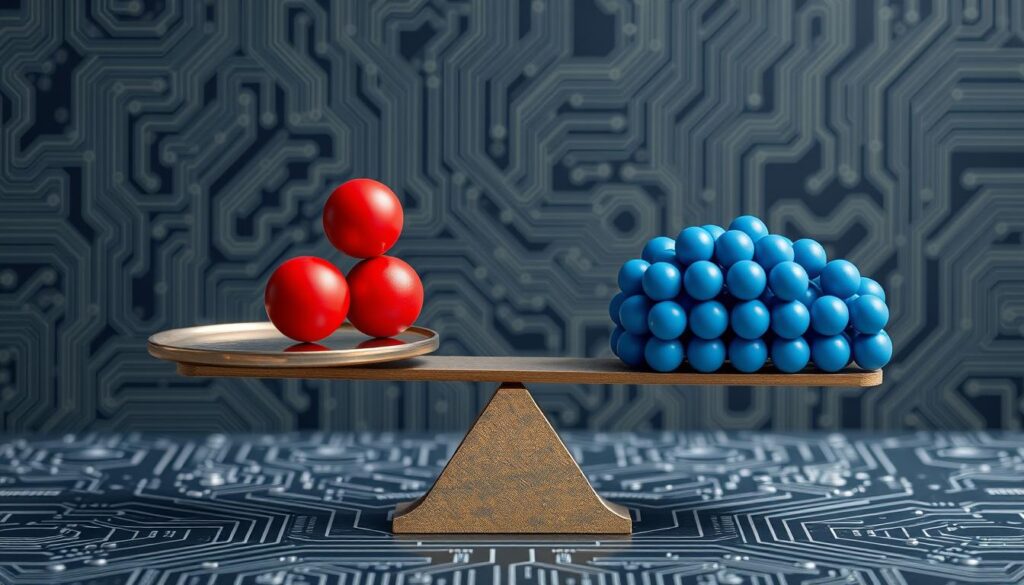
It’s crucial to tackle imbalanced datasets to create reliable machine learning models. These models should perform well across all classes, especially the minority class, which is often more important in certain scenarios.
Why Imbalanced Data Creates Problems in Machine Learning
Imbalanced datasets are a big challenge in machine learning. When there’s a huge difference in the number of classes, models can become biased. This means they often favor the majority class, leading to poor performance on the minority class.
Traditional metrics like accuracy can be misleading with imbalanced data. Even if a model seems accurate, it might not do well on the minority class. This is a big problem in areas like fraud detection or disease prediction, where getting it wrong can have serious consequences.
For instance, in fraud detection, most transactions are genuine, but a small fraction is fraudulent. In ad serving, most data is about non-clickers, while only a few instances are about clickers. In these cases, models tend to favor the majority class, making it hard to spot the minority class.
Imbalanced data affects different machine learning algorithms in different ways. Some, like Logistic Regression and Naive Bayes, tend to favor the majority class. But decision tree-based models are less affected, as they consider all classes during splitting.
To tackle imbalanced data, techniques like oversampling, undersampling, and advanced methods like SMOTE are key. These methods help balance the class distribution. However, they also come with risks like overfitting or underfitting.
In summary, imbalanced data is a major issue in machine learning. It needs careful handling and the right techniques to ensure models perform well and accurately, especially in critical areas where the minority class matters most.
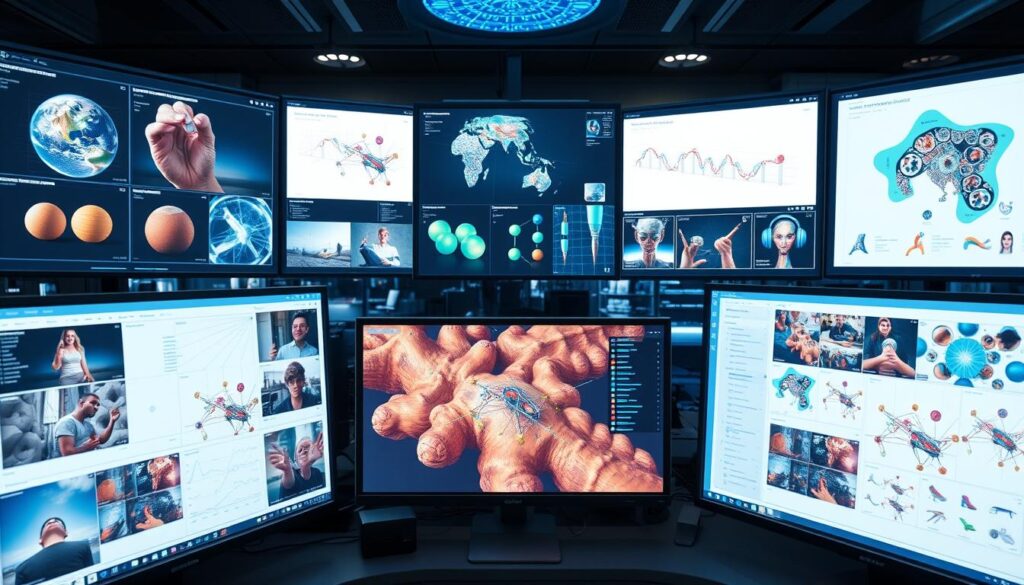
Essential Techniques for Handling Imbalanced Data
In Machine Learning, Artificial Intelligence, and Data Mining, dealing with imbalanced datasets is a big challenge. These datasets have one class much larger than the others. This can make models biased and predictions inaccurate. Luckily, there are key techniques to tackle this problem and boost model performance.
Random Undersampling and Oversampling Methods
One way to handle imbalanced datasets is through random undersampling and oversampling. Random undersampling reduces the majority class by removing observations. This makes the class distribution more balanced. On the other hand, random oversampling increases the minority class by duplicating observations. This boosts the minority class’s representation.
SMOTE and Its Variants
The Synthetic Minority Oversampling Technique (SMOTE) is a popular method for imbalanced datasets. It creates synthetic examples of the minority class by finding k-nearest neighbors. New samples are made along the lines connecting minority class instances. Variants like BorderlineSMOTE and SVM-SMOTE focus on samples near decision boundaries to enhance model distinction.
Advanced Resampling Techniques
There are also advanced resampling techniques for imbalanced datasets. These include clustering the majority class to find and remove outliers. Variations in synthetic samples for the minority class are introduced to improve generalization. The imbalanced-learn Python library offers these advanced methods.
By using these essential techniques, machine learning experts can overcome class imbalance challenges. This leads to more accurate and reliable models in Machine Learning, Artificial Intelligence, and Data Mining.
Technique | Description | Advantages | Disadvantages |
---|---|---|---|
Random Undersampling | Reducing the size of the majority class by randomly removing observations | Simple to implement, can improve model performance | May result in loss of valuable information from the majority class |
Random Oversampling | Increasing the size of the minority class by duplicating existing observations | Can improve model performance on the minority class | May lead to overfitting due to duplication of instances |
SMOTE | Generating synthetic examples of the minority class by computing k-nearest neighbors | Preserves the distribution of the minority class, can improve model performance | May create unrealistic synthetic samples, require careful parameter tuning |
Advanced Resampling Techniques | Clustering the majority class, introducing variations in synthetic samples | Can address more complex imbalance scenarios, provide better generalization | Require more computational resources, may be more complex to implement |
Evaluation Metrics for Imbalanced Dataset Classification
When working with Predictive Analytics, Supervised Learning, and Deep Learning, accuracy metrics can be tricky. This is especially true for imbalanced datasets. To get a clear picture of how well these models perform, we need different evaluation metrics.
The F1 score is a key metric here. It’s the average of precision and recall. This score helps us see how well the model does on both common and rare classes. It’s very useful for data that’s not evenly distributed.
The area under the ROC curve (AUC-ROC) is also important. It shows how well the model finds true positives without false positives. But, for very imbalanced data, the precision-recall curve is more accurate.
Other metrics like weighted balanced accuracy, normalized inverse class frequency, and probabilistic F score are also helpful. They adjust accuracy based on class weights and consider prediction confidence. These metrics give a fuller view of the model’s performance.
Choosing the right metrics is key to understanding how well a model works. It helps us improve how we handle imbalanced datasets in Predictive Analytics, Supervised Learning, and Deep Learning.
Metric | Description | Performance |
---|---|---|
Weighted Balanced Accuracy | Adjusts the accuracy metric based on class weights, giving higher weightage to classes with lower class weights. | Reaches its optimal value at 1 and its worst value at 0. |
Normalized Inverse Class Frequency | Commonly used for setting class weights in imbalanced data scenarios. | The weighted balanced accuracy reaches its optimal value at 1 and its worst value at 0. |
F-beta Score | Provides a weighted harmonic mean between precision and recall, with beta values determining the weight given to recall. | High scores indicate accurate results and a majority of positive results being returned. |
Probabilistic F Score | Accepts probabilities instead of binary classifications, is less likely to produce NaN values, and is sensitive to the model’s prediction confidence scores. | Provides a more comprehensive assessment of the model’s performance. |
Precision-Recall Curve | Measures relevancy and precision among retrieved instances. | High scores indicate accurate results and a majority of positive results being returned. |
AUC-ROC Curve | Measures the trade-off between true positive rate and false positive rate. | Not the preferred metric for severe imbalances as it can lead to unrealistic results in scenarios with a very small number of rare classes. |
Conclusion
Dealing with imbalanced datasets is a big challenge in machine learning and data science. Techniques like resampling and special algorithms help. They make models better at spotting both common and rare patterns.
A new method called ‘prediction-powered inference’ shows great promise. It uses lots of machine learning guesses and a little bit of real data. This way, it checks if the predictions are reliable.
As machine learning grows, so does the need to handle imbalanced data. Learning these methods is key. It helps businesses get the most out of machine learning. This leads to better results in finance, healthcare, and more.